The Case for Model Disgorgement: Why AI “Art” Is Not Transformative
The function of the transformer in ChatGPT seems akin to putting together a billion drops of water . . . some from the Ganges, some from the Nile, some from the Mississippi, some from La Seine . . . putting random drops of water in a tub and claiming I have transformed water itself into . . . water.
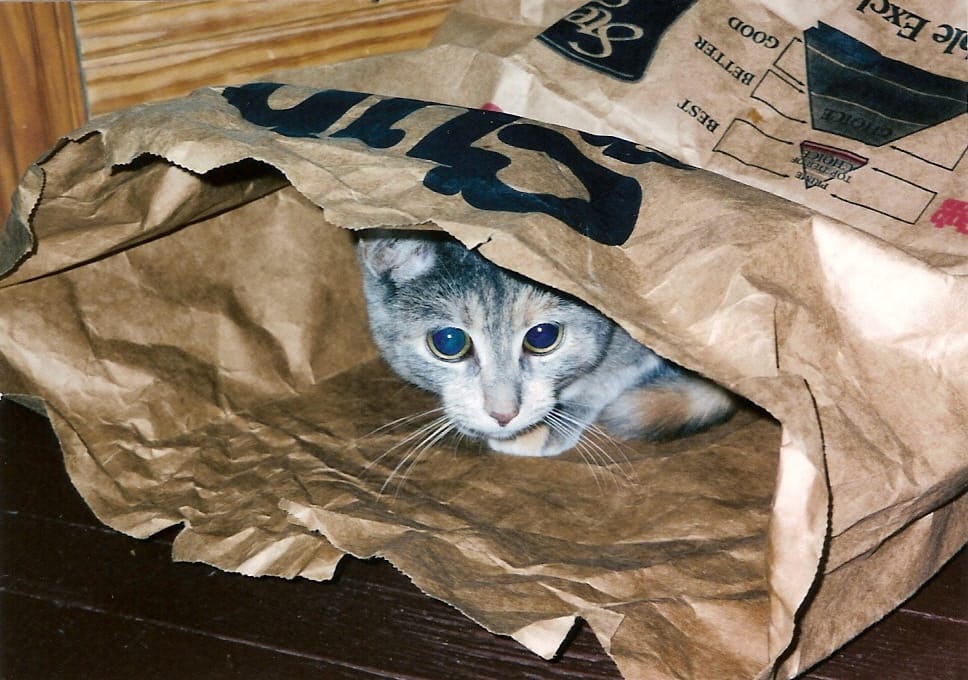
*Note that neither this nor any Artists Resisting Exploitation article should be construed as legal advice.
What is transformative? We may, for reasons “unknown” (basically, greed), find ourselves oddly unable to put the cat back in the bag every cat owner knows it normally pops in and out of or the genie back in the bottle/lamp that, aside from Disney’s Americanized reimagining of an ancient Arabic folktale, it’s lived in for centuries and to which it usually returns after every wish—in which it is literally imprisoned—but we can at least stuff the rabbit back down the black box, I mean hat, from whence it came. Let’s unpack the concept of transformation as it applies to AI-generated text, images, audio, and video.
First, let’s attack this from the most pertinent angle: the law. In their analysis of what the recent Supreme Court decision in Andy Warhol Foundation for the Visual Arts, Inc., Petitioner v. Lynn Goldsmith, et al. means, international law firm Mayer Brown writes, “The Supreme Court explained that the Supreme Court’s previous decision in Campbell cannot be read to mean that any use that adds new expression, meaning, or message is a ‘transformative’ fair use. Otherwise, ‘transformative use’ would eviscerate the copyright owner’s exclusive right to prepare derivative works, as many derivative works add new expression of some kind” (2023, emphasis added). This limits the scope of fair use and clarifies that the Supreme Court may consider additional pertinent information in determining whether something presented as “fair use” is actually copyright infringement.
However, it’s important to note that “transformative” is itself fairly ill-defined legalese that appears to be largely dependent on the interpretations of individual judges. This is problematic because if there was ever a time to clarify, once and for all, what is and isn’t transformative, this—a time when creative industries are in danger of being decimated by generative AI models that were created using unlicensed copyrighted content that is then used to produce similar content—would be it. Note, also, that, as law scholar Ben Sobel writes in his brilliant analysis Elements of Style: Copyright, Similarity, and Generative AI, the notion, popular with AI companies and their supporters, that “you can’t copyright style” is “at best, meaningless”and “more likely, it’s wrong”(2024). Style, Sobel, asserts, is sometimes copyrightable. Even if the similarities fall under what some may consider “style,” a work that shows “substantial similarity” to a pre-existing copyrighted work can, nevertheless, be deemed to infringe on the copyright of the original work. The fact that judges find it difficult to determine “substantial similarity” in art (especially visual art) further muddies the waters.To prevent occurrences like the current crisis artists face,which has been manufactured by generative AI companies and which could be brought about again by future corporations and sycophants who seek to profit from exploiting artists,we need to arrive at a consensus of what is not protected by fair use and, in particular, by the “transformative use” clause.What might a court consider in clarifying, once and for all, what “transformative” means?
According to Merriam-Webster, “transform implies a major change in form, nature, or function” (2024). To further clarify what this means, “nature,” again according to Merriam-Webster, refers to “character or constitution” (2024). Therefore, transformation requires significant alterations in the form, makeup of, and/or function of something.
The truly radical transformations that we’ve experienced as a species include the ability to conduct electrical charges in order to make them useful and the ability to digitize knowledge, ideas, skills, actions, and one of their manifestations: art. Despite what profiteers may claim, generative AI in the arts is not transformative. It does not significantly alter the “form, nature, or function” of art to create anything new but is, essentially, the world’s largest kaleidoscope, rearranging what exists in patterns that may appear novel but, all too often, infringe on existing works. Indeed, in its explanation “What is generative AI and how does it work?,” Adobe, which is as much of an AI company now as Meta or Amazon, likens generative AI to “a kaleidoscope that makes a familiar view fresh” (2024).
CNBC’s Hayden Field reports that, according to responsible AI researcher Rebecca Quian of Patronus AI, “We [Patronus AI] pretty much found copyrighted content across the board, across all models that we evaluated, whether it's open source or closed source.” Moreover, “Perhaps what was surprising is that we found that OpenAI's GPT-4, which is arguably the most powerful model that's being used by a lot of companies and also individual developers, produced copyrighted content on 44% of prompts that we constructed" (2024, emphasis added). This is a far greater percentage of copyright infringement than generative AI companies and supporters admit to. This reality also supports this article’s central claim: AI models/companies and their users merely turn art into more art in the same discipline and/or genre and which often looks or sounds similar to the existing works on which generative AI models were trained. This is not transformative.
As OpenAI’s James Betker noted on his blog, “It’s becoming awfully clear to me that these models are truly approximating their datasets to an incredible degree.” Betker added, “It implies that model behavior is not determined by architecture, hyperparameters, or optimizer choices. It’s determined by your dataset, nothing else” (2023, emphasis added). Generative AI outputs do not meet the basic standard definition of transformative because neither the form, nor the makeup, nor the function of the art is significantly altered. All of the value added in generative AI comes from the works of artists and other copyright owners whom generative AI companies would like to avoid fairly remunerating while perversely claiming that this stolen data is the AI corporations’ or startups’ intellectual property and their “trade secrets” in a twisted bid to avoid the data transparency that would enable copyright owners—the actual rightful owners of that data—to see what’s inside generative AI black boxes and, thus, make it easier for copyright owners to prove infringement.
On the output side, a user may prompt a model a hundred times to produce a result that is less, to borrow from artist Kelly McKernan,“infringe-y,” but this does not negate the initial infringing output, which is where the focus should be since there is no guarantee that every user will dedicate time and attention to altering outputs and it is much more likely that most won’t. Moreover, future outputs that build on the initial generation may be considered separate works, enabling a user to infringe on artists’ copyrights multiple times until arriving at a result that most would consider different enough. This user can then sell all of the generations as, say, a series rather than discarding any of them. For these reasons, every time the user outputs an image, audio, video, or text, a new analysis of whether that particular output infringes on copyrights should be considered. We are not concerned with what the user does after using the AI (whether the user alters the image in Photoshop, for example) but with what the AI generates at any given time. We cannot rely on users being moral/ethical and must, in fact, plan for neutral users who do nothing but accept what the AI generates at face value. Further complicating this is the fact that AI models can produce substantially similar work for multiple users. According to Suno AI’s Terms of Service, “Due to the nature of machine learning, Output may not be unique across users and the Service may generate the same or similar output for a third party”(2024).
AI companies bear the brunt of responsibility for the lack of actually transformative work their models generate because they take unnecessary risks by using copyrighted unlicensed content in training data, which can then produce outputs that further infringe on artists’ copyrights. Crucially, even generative AI supporters like Professor Murray admit that training generative AI requires creating a temporary copy of each work. He writes, “Examples of this are incidental or intermediate processing of data from expressive works that requires the works to be downloaded (which in the digital context means a copy of the digital work necessarily was made) or it is copied in the functional process of analysis.” However, Murray argues, this “does not count as an ‘act of copying’ or it is excused from infringement by the fair use doctrine” because the original artworks are being “[transformed] into machine readable numeric data” only for the purposes of training the AI (2023, 14–18). Never was a more winding and lawyerly justification for a crime composed. Sobel soundly refutes this. He writes:
The logic that allows Vincent [and, I would add, Murray] to claim that AI models don’t “store images” also dictates that laptop computers don’t “store images” either. If you pry open your laptop, you won’t find a sheaf of images in there – no matter how many pictures you have saved on your hard drive. A digital photo library is a collection of bytes that instructs a computer to display particular images under particular circumstances. Conventional image file formats are just “mathematical representations of patterns” that appear in the images to which they correspond. An image file might instruct a computer, “display a 50x50 pixel grid of alternating rows of white and red pixels.” That’s a mathematical representation of a white-and-red-striped square. It’s just a mathematical representation that happens to be more comprehensible to us than latent images in a diffusion model are. (23–24)
Sobel concludes, “Once we acknowledge that all digital images are mathematical representations of visual phenomena, it becomes impossible to distinguish between ‘piec[ing] together bits of images’ and ‘creat[ing] pictures from scratch’ unless we can explain why we ought to treat some mathematical representations differently from others” (24, emphasis added). Just as they downplay the extent to which their models output infringing content, generative AI companies and their supporters downplay the reality that the temporary copies they must make in order to gather and process data to train foundational models also constitute copyright infringement.
Generative AI companies and their supporters go further, claiming that even if this is copyright infringement, it should be allowed since (a) it enables new artistic works to be created and (b), according to Sam Altman, Andreessen Horowitz, and others who profit from the exploitation of artists, it’s “impossible” to build generative AI models without using unlicensed copyrighted works. As if to justify this, Murray writes:
To the extent that the transformation of images into machine readable numeric data is found to be a form of copying, it is a machine function that allows the users of the machine to express themselves through the generation of new and original images. The machine’s function is copy-reliant on learning what images of various kinds look like by training on hundreds of millions or billions of images, but the copying of image data serves a function and purpose of building machine systems that enable new artists to create original artistic expression. (18)
Both of the two arguments put forward to justify using unlicensed copyrighted content to train generative AI are preposterous in light of (a) the degree to which it is questionable that users of generative AI can be credited as creators of AI outputs, (b) the extent to which the originality of those outputs is also questionable, and (c) the existence of various ethically trained generative AI models that were created by licensing content (such as those found at Fairly Trained’s website) and the other ethically trained models that are emerging from licensing agreements between AI companies and owners of copyrighted works (for example, the recent deal between Universal Music Group and SoundLabs).
I cannot steal millions of Ford, Tesla, and Toyota cars to create a machine that outputs hybrid vehicles—let’s call them Fortes’ Toys—that can be reconfigured based on customer preferences and get away with this. I especially cannot do this when many of these hybrid cars bear semblances to the original Ford, Tesla, and Toyota cars I stole—when my somehow respectable scam masquerading as an actual business is, essentially, entirely reliant on the brand recognition, reputations, quality products, and other strengths of other businesses without which my business would be nonexistent yet which I, essentially, bleed dry. Again, as I wrote in “When the People Say No Mo’: Waymo Vandalism as a Human Reaction to Techsploitation,” if we privilege material property over intellectual property, then we exacerbate economic inequity and inequality and we severely limit socioeconomic mobility since those who have the most material property—those who are already wealthy—gain even more power over those who do not.
One wonders whether AI developers—many of whom, despite the irony of now being almost entirely beholden to the written word for the usefulness and success of their products, often have more reverence for math than for the written word (who too often lack respect for the humanities and, in too many cases, for actual humanity)—latched onto the word “transformer” and assumed that this would suffice as proof of generative AI’s “transformativeness.” The T, after all, in ChatGPT stands for “transformer.” A transformer moves energy from one circuit to another or to other circuits while increasing or decreasing the voltage of that energy. Note that a transformer does not significantly alter the form, nature, or function of the energy itself. It merely reduces or increases the amount of energy that is transported from one point to another. The function of the transformer in ChatGPT seems akin to putting together a billion drops of water—some from the Red Sea, some from the Black; some from the Pacific, some from the Atlantic; some from a sewer in South London; some from wells in Arkansas and Oregon; some from the Three Gorges Dam; some from an apartment toilet in Seoul, some from a restroom in a strip mall outside of Boston; some from an actual strip club; some from the Ganges, some from the Nile, some from the Mississippi, and some from La Seine; some from condensation on an apartment window in San Francisco’s Mission District; some from droplets on a fern in the Amazon rainforest; and topping it off with melting Arctic glaciers—putting all these random drops of water in a tub and claiming that I have transformed water itself into . . . water.
“But,” an AI company or prompt engineer might argue, “We now have more water for everyone! This is democratization!” I would counter that giving all the world’s water to corporations, mixing all of it—some of which was pure and some of which was undrinkable—in order to have a greater total volume of water that is polluted to a level deemed acceptable for human consumption by purely profit-motivated, unregulated corporations is counterproductive, especially when the process of creating this lower-quality but greater quantity of water consumes vast amounts of extremely precious fresh water like the data centers required to power generative AI do. I would insist that precious fresh water belongs to the people—often indigenous, but nearly always local, people—who’ve stewarded the environments that keep that water as fresh and as abundant as possible. Data sovereignty means it is those long-time stewards of those sources of fresh water who should decide how it is shared. This is even more true when the “water” in question is, essentially, the sweat, blood, and tears of artists themselves.